Since the beginning of 2023, my Foundamental colleagues and I have seen a huge inflection of tech founders and startups applying Neural Radiance Fields (NeRFs) to built-world objects.
Get the slide deck
NeRFs are state-of-the-art AI networks to generate 3D representations of an object or scene from a partial set of 2D images. For example, they even get reflections correctly represented in 3D. In a dumbed-down way, you can think of NeRFs as taking a 3D scan with just a couple of 2D photos from different angles.
Basically, NeRFs killed photogrammetry. (I won’t be writing about the technology behind NeRF’s in this post. You can read more about NeRFs in more detail here, here and here.)
This post is about my hypotheses what opportunities NeRFs create, for example in the building-world.
It’s an early and evolving post as my understanding of the applications of NeRFs and the market evolve. So I might update the post over time.
10 theses on big opportunities that NeRFs create, as of July 2023
Starting with a first principle bedrock: Through NeRFs, hundred of billions of objects and scenes will get captured. Because NeRFs in itself are open-source and easily adoptable, the NeRF tech will not be defensible nor differentiated in itself. The sheer scale of capturing of hundreds of billions of assets, however, will create new frictions in the 3D tool stack, and thus massive new opportunities.
Here are 10 current theses I play around with thinking about where value can get created in the context of massive adoption of hundreds of billions of NeRF-based assets.
Quick definition: In the context of this thesis, by “asset” we refer to 3D models, scenes and textures alike.

Upstream
Billions of new assets: NeRFs will capture and encode hundreds of billions of real-world objects and scenes in a matter of a few years.
Capturing is commodity: The upstream capturing and encoding with NeRFs will be commodity fast, and not sufficiently differentiated.
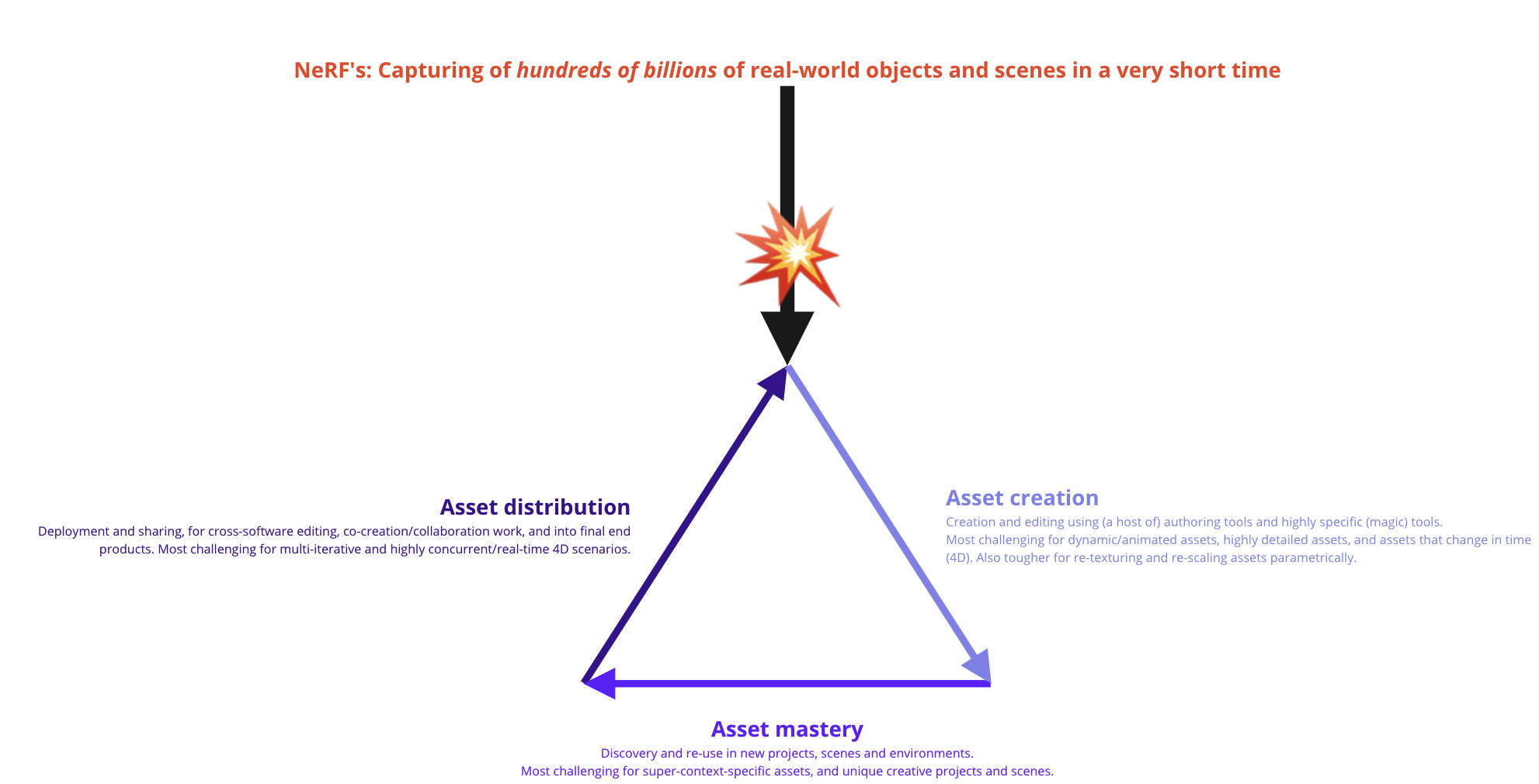
Asset creation
- More everyday authoring: The legacy 3D tool stack requires thousands of hours of practice to achieve routine and excellence. As 3D becomes accessible to new generations and user groups, modern authoring software built on concurrency (unlocking multi-player collaboration) and versioning reduces the entry barriers for casual 3D creators, and improves the integrations and open-ness for professional creators.
- Reconstruction-as-a-process: Parametric is a thing, but it follows pre-determined rules and requires significant customization to the model or the scene. To animate a NeRF’ed object, or to re-texture it, or to change key aspects (eg. a chair with 3 instead of 4 legs), the tool-stack will require pipelines to AI-trained engines that automatically can upgrade NeRF’ed objects into new motion, texture or parametric changes. This shall include automated animation using trained models. It’s to be determined if this might require an infrastructure/API type deployment to make use of very advanced 3D infrastructure for the parametric system.
- Magic tool-suites: A modern authoring tool is best when it does NOT try to fulfill every specialty function. That’s where magic tool suites come in. They are suites of ultra specialized functions, features and algorithms, which are interoperable with the authoring tool of choice.
Mastery
- Decreasing marginal value, unless >>> Segmenting and tagging of real-world items: The flood of 3D’d real-world content could decrease the marginal value of access to yet another 3D asset, as discovery becomes a problem with every asset added. That’s why segmenting and tagging need to be automated. These are trained models to classify 3D assets on various vectors. While easier on everyday items that are google-able, it becomes an ULTRA technical problem and opportunity on non-googleable asset training data, eg. industrial assets or special mechanical parts of an object.
- A new Digital Twin Management / PIM stack (especially for reconstructed built-world objects): To organize the discovery and re-use of assets via AI, new 3D product information management (PIM) software will be required to store, share and re-use (reconstructed) assets easily.
Distribution
- 4D NeRF streaming: This is a thesis I am currently most intrigued by. A true moonshot. Imagine a sports event, or a frontline defense use case, where objects and scenes get captured and encoded as the objects and scenes move through time (4D) and not re-live, but live. And those objects and scenes get encoded in (near) real-time into a 4D experience (eg. via an AR such as Apple’s Vision Pro headset) to the user. This moonshot, if feasible, requires an infrastructure layer or service to receive, encode and send back real-time NeRFs moving through time into live experiences for events, defense and actually any remote real-world collaboration.
- E-commerce deployment: While this is a thesis many folks seem to think about lot, and I find actually really boring for its crass consumerism. It means the deployment of products in their digital 3D representation into 3D shopping environments. While I get the market, it should be noted it will require significant hardware penetration before inflection.
- Full-stack consumer suite: Think Instagram, but for NeRF’d content. Capture-edit-distribution via a mobile phone app + community. A very smart founder told me: “Our phone screens are sadly 2D”. Something to think about.
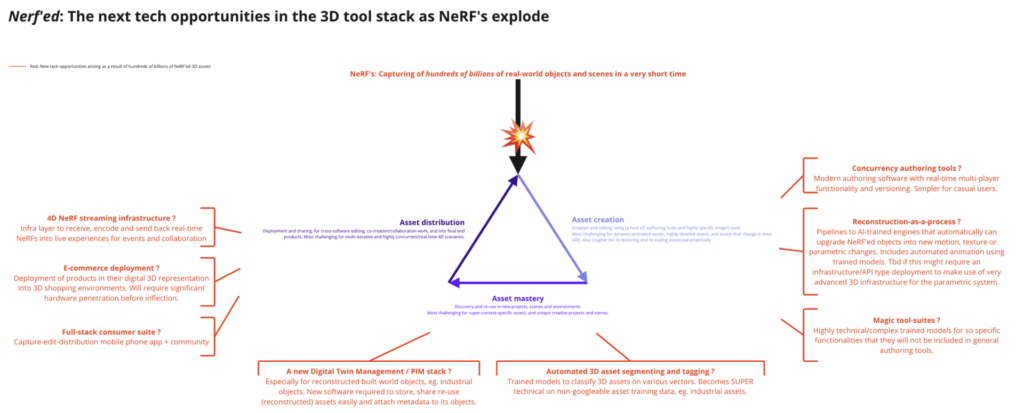
Unclear to me how it will play out in the market
- AI animation – on what data: NeRF’d objects are static (today). Creating animated 3D content is insanely complex and a mix of science, craftsmanship and art. Animators deserve every penny they get paid. But there’s just not that many of them. Now, how do we animate content from a static NeRF to a dynamic model and scene with AI? It’s actually a data availability problem. My bet is you will never have as much animated content to train on than you will have static content. Thus, one scalable solution might be to train on deducing movement from static content. We’ll see.
- NeRFs as shells: As NeRF’d content (today) will capture the surface of objects, but not its inner contents, it creates billions of shell models, not solid models. I have yet to figure out what this fact will do with its scaling potential in which segments.
- Attaching dimensions to NeRF’ed objects: I have yet to see a robust approach that attaches dimensions (cm, mm, inch) to NeRF’d models. Can anyone point me to a robust approach?
Have thoughts on my thoughts? I’d love to hear yours.